
Significant advances in quantitatively understanding such complexity have been registered in recent years, as those involving the growth of cities but many fundamental issues still defy comprehension. Millions of individual decisions, motivated by economic, political, demographic, rational and/or emotional reasons underlie the high complexity of demographic dynamics. The plots below show how the cost relates to our prediction (the first plot depicts how cost changes relative to our prediction when the Actual Outcome =1 and the second plot shows the same but when the A ctual Outcome = 0).Understanding demographic and migrational patterns constitutes a great challenge. The model was pretty sure that I would miss and it was wrong so we want to strongly penalize it we are able to do so thanks to taking the natural log. In this case we are massively wrong and our cost would be: Now let’s pretend that we built a crappy model and it spits out a probability of 0.05.But the model was not 100% sure that I would make it and so we penalize it just a little for its uncertainty. Assuming the default cutoff of 50%, the model would have correctly predicted a 1 (since its prediction of 95% > 50%). Also, I want to emphasize that this error is different from classification error. Notice how close it is to just taking the difference of the actual probability and the prediction. The penalty in this case is 0.0513 (see calculation below). But it was only slightly wrong so we want to penalize it only a little bit. So the model was wrong because the answer according to our data was 100% but it predicted 95%.In the actual data, I took only one shot from 0 feet and made it so my actual (sampled) accuracy from 0 feet is 100%.Let’s say it estimates 0.95, which means it expects me to hit 95% of my shots from 0 feet. First my model needs to spit out a probability.Yay, I don’t completely suck at basketball. In my basketball example, I made my first shot from right underneath the basket - that is. Don’t despair, we can do something very similar. But here we are dealing with a target variable that contains only 0s and 1s. This is easy to visualize in the linear regression world where we have a continuous target variable (and we can simply square the difference between the actual outcome and our prediction to compute the contribution to cost of each prediction). So if my prediction was right then there should be no cost, if I am just a tiny bit wrong there should be a small cost, and if I am massively wrong there should be a high cost. A cost function tries to measure how wrong you are. So let’s first start by thinking about what a cost function is.

Like most statistical models, logistic regression seeks to minimize a cost function. Odds as you can see below range from 0 to infinity.

So if they basically tell us the same thing, why bother? Probabilities are bounded between 0 and 1, which becomes a problem in regression analysis.
#Logistic fx equation explination free
My odds of making a free throw can be calculated as: Based on this sample, my probability of making a free throw is 70%. Odds is just another way of expressing the probability of an event, P(Event).Ĭontinuing our basketball theme, let’s say I shot 100 free throws and made 70. Now unless you spend a lot of time sports betting or in casinos, you are probably not very familiar with odds. However, in logistic regression the output Y is in log odds.
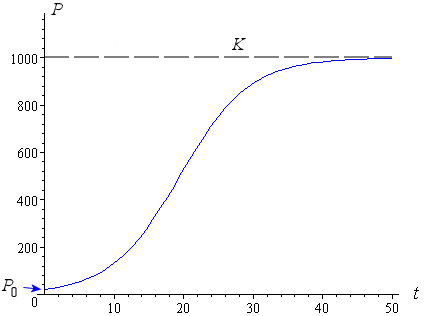
In linear regression, the output Y is in the same units as the target variable (the thing you are trying to predict). So let’s start with the familiar linear regression equation: So we can already see the rough outlines of our model: when given a small distance, it should predict a high probability and when given a large distance it should predict a low probability.Īt a high level, logistic regression works a lot like good old linear regression. Generally, the further I get from the basket, the less accurately I shoot.
